How to Automate Lead Qualification and Segmentation with Enriched Data
Lead qualification and segmentation: what it is and why it's essential for your sales success
Blogby JanApril 20, 2025
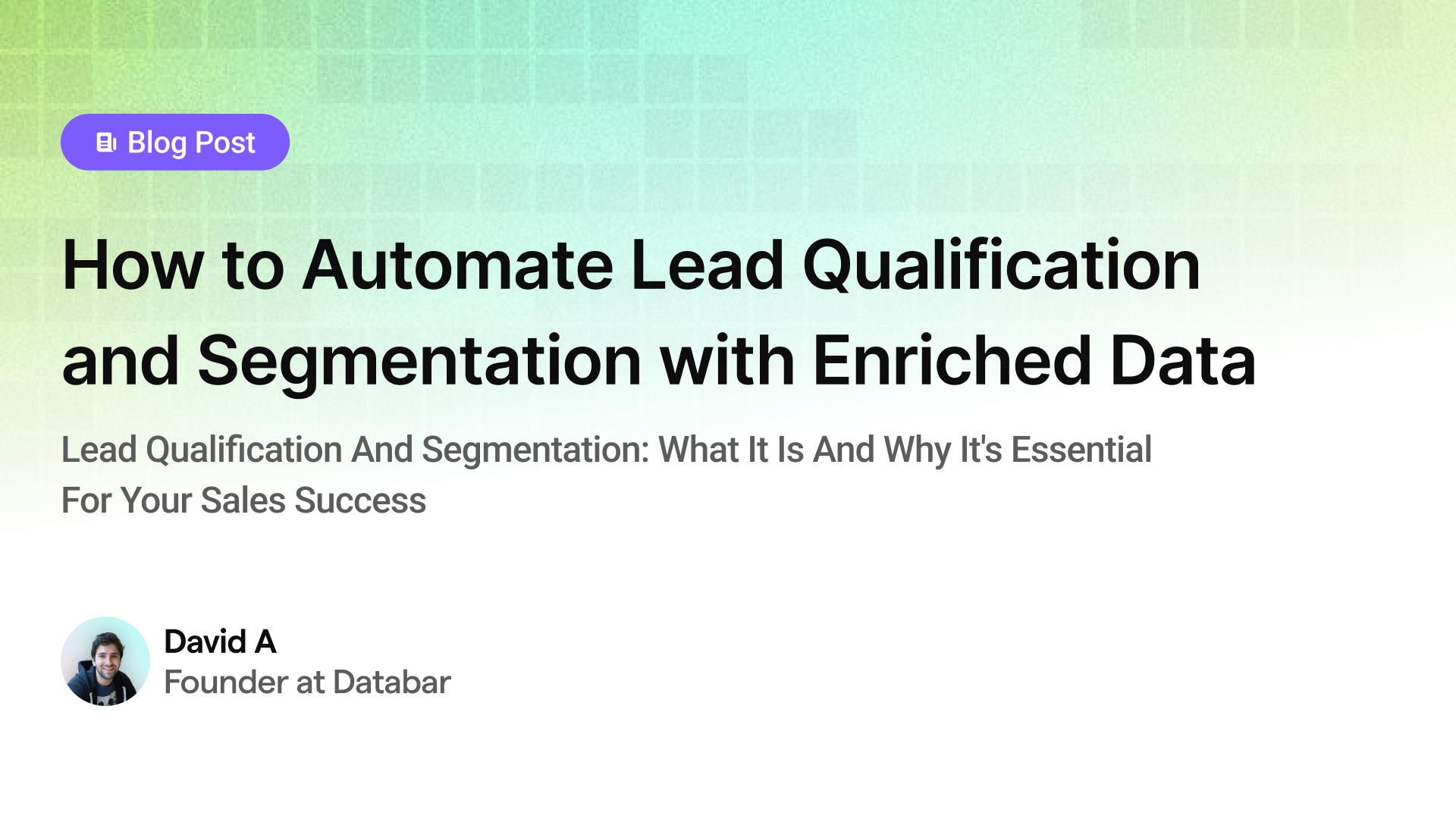
If you've ever sent the same email to 500 leads only to get 1 lukewarm response, you know the pain of poor segmentation. Mass outreach without proper qualification is like fishing with dynamite – destructive, inefficient, and likely to leave you empty-handed.
Sales teams that drown in "leads" while starving for customers share a common problem. They treat wildly different prospects as if they were identical. The VP of Engineering at a Fortune 500 company doesn't have the same pain points, buying process, or decision criteria as a startup founder – so why would anyone approach them the same way?
Lead qualification and segmentation aren't just nice-to-haves anymore. Companies that effectively segment their prospects see conversion rates up to 355% higher and revenue increases of 781% compared to those using basic or no segmentation.
This guide will walk through exactly how to automate lead qualification and segmentation using enriched data – from basic techniques to advanced workflows that can transform outreach results practically overnight.
Why traditional lead qualification methods fail
Let's be honest about traditional qualification methods. They worked in a different era – when information was scarce, buyers had fewer options, and the first salesperson to make contact often won the deal.
Today's reality? Prospects are drowning in outreach. They're researching solutions long before they talk to sales. And they expect you to understand their specific situation without them having to explain it.
Manual qualification based on gut feeling or superficial criteria just doesn't cut it anymore. When sales reps qualify leads based on "feel" or basic firmographic data, they miss critical signals that indicate buying intent or fit. They waste time on prospects who will never buy and overlook those who actually need the solution.
Organizations often spend months chasing leads at companies that match their ideal customer profile on paper – right industry, right size, right titles – but have just implemented a competitor's solution. That's months of wasted effort that could be avoided with proper data enrichment showing recent technology purchases.
The alternative? Data-driven qualification that combines multiple signals to create a complete picture of each prospect. This is where enriched data becomes a secret weapon.
Understanding enriched data for qualification
Enriched data goes beyond basic contact information to provide context about who leads are, what challenges they face, and how likely they are to need a solution.
Think of it this way: basic lead data might tell you someone's name, title, and company. Enriched data tells you their technology stack, recent funding rounds, growth trajectory, pain points, competitive landscape, and buying signals – all the information needed to determine if they're a good fit and how to approach them effectively.
Here are the key types of enriched data that power effective lead qualification:
Firmographic data provides company-level information like industry, employee count, revenue, location, and founding date. This helps determine if a company matches the ideal customer profile at a basic level.
Technographic data reveals the technologies and tools a company uses. This is crucial for understanding if they use complementary solutions, competitor products, or have the technical infrastructure a product requires.
Intent data tracks research and buying behaviors, showing which companies are actively looking for solutions. This includes website visits, content downloads, webinar attendance, or third-party research on related topics.
Engagement data measures how prospects interact with content and communications. This includes email opens, link clicks, meeting attendance, and other signals of interest.
Demographic data provides individual-level information about contacts, including job responsibilities, tenure, career history, education, and personal interests.
Behavioral signals track actions that indicate buying readiness, such as pricing page visits, free trial usage patterns, or specific feature inquiries.
When combined, these data points create a multidimensional view of each prospect that allows for precise qualification and personalized outreach that resonates with their specific situation.
Building your automated qualification workflow
Creating an automated qualification workflow doesn't happen overnight, but the payoff is enormous. Here's a step-by-step approach to building a system that consistently identifies and prioritizes the best leads.
Step 1: Define your qualification criteria
Before starting automation, there needs to be clarity on what makes a qualified lead for the business. This means defining an ideal customer profile (ICP) with specific, measurable criteria.
Working backward from the best customers makes sense. Looking at the 20% of customers who generate 80% of revenue or success stories reveals shared characteristics. What problems were they solving when they purchased the solution?
Documenting these criteria in detail and converting them into measurable data points is essential. For example, instead of "companies that need better customer service," define it as "companies with over 10,000 customers and fewer than 50 support agents."
Make sure criteria include both positive indicators (signals the prospect is a good fit) and negative indicators (signals they're unlikely to buy). Both are equally important for accurate qualification.
Step 2: Map your data enrichment needs
Once the qualified lead profile is established, the next step is identifying what data is needed to evaluate each criterion. Then determining how to source that data consistently and at scale.
For example, if one criterion is "using competitor technology," technographic data from a provider that tracks software installations or technology mentions will be needed. If "recent funding" matters, financial data that's updated frequently becomes important.
Creating a comprehensive data map that includes:
- Each qualification criterion
- The specific data points needed to evaluate it
- Potential data sources for each point
- How frequently the data needs to be refreshed
- Relative importance of each criterion in the overall qualification score
This map becomes the blueprint for automated qualification, ensuring all necessary data for accurate assessment is collected.
Step 3: Set up your data enrichment pipeline
With data needs mapped, it's time to build the enrichment pipeline that will feed the qualification system. This is where platforms like Databar.ai become invaluable.
Databar.ai connects to 100+ data providers through a single interface, allowing lead enrichment with all needed information without juggling multiple tools or APIs. The platform's custom enrichment workflows let users define exactly what data to collect for each lead and how to process it.
For example, a workflow might:
- Take a list of company domains from a CRM
- Enrich them with firmographic data from provider A
- Add technographic data from provider B
- Incorporate intent signals from provider C
- Verify and standardize all information
- Push the complete dataset back to the CRM
What makes Databar.ai particularly powerful is its waterfall enrichment capability. Instead of relying on a single source for each data point, the system automatically tries multiple providers in sequence until it finds high-quality information. If the first provider doesn't have the particular data for a company, it seamlessly moves to the next provider, and the next, until it finds what's needed.
This approach dramatically increases data coverage and accuracy, especially for niche industries or smaller companies that might not be well-represented in some databases.
Step 4: Create your lead scoring model
With enriched data flowing in, the next step is creating a scoring system that automatically evaluates each lead against qualification criteria.
The most effective lead scoring models combine:
Fit score – How well the company matches the ideal customer profile based on firmographic and technographic data. This measures whether they could buy the solution.
Intent score – How actively the company is researching solutions based on intent and behavioral signals. This measures whether they're ready to buy.
Engagement score – How responsive the company has been to outreach efforts. This measures whether they're interested in buying from you.
Each criterion in the model should have a clear point value based on its predictive power. For example, using complementary technology might be worth 15 points, while being in the target industry might be worth 10 points.
Setting threshold scores for different qualification levels makes sense:
- 85+ points = Sales-ready lead
- 60-84 points = Marketing-qualified lead
- 40-59 points = Nurture-stage lead
- Below 40 = Unqualified
These thresholds will evolve as more data is gathered, but they provide a starting point for automated routing and prioritization.
Step 5: Implement custom industry segmentation
One of the most powerful applications of enriched data is custom industry segmentation that goes beyond standard classifications. This is where Databar.ai's AI-powered classification really shines.
Standard industry categories from data providers are often too broad or inconsistent for effective targeting. For example, "Healthcare" might include hospitals, health insurance companies, medical device manufacturers, and healthcare software providers – all with vastly different needs and buying processes.
Using Databar.ai, teams can create custom industry segments tailored to their specific market approach. The platform uses AI to analyze company descriptions, websites, and other data points to place each prospect into custom-defined segments.
For example, a cybersecurity solutions company might use Databar.ai to create custom segments like:
- Financial Services: Banks
- Financial Services: Insurance
- Financial Services: Investment Management
- Healthcare: Hospitals
- Healthcare: Pharmaceutical
- Healthcare: Medical Devices
- Technology: SaaS
- Technology: Hardware
This granular segmentation allows for highly targeted messaging addressing the specific security concerns and compliance requirements of each sub-industry.
The process works by:
- Creating clear definitions of each custom segment
- Feeding company descriptions and website content into the AI classification engine
- Automatically categorizing each company into the appropriate segment
- Applying segment-specific qualification criteria and messaging approaches
The best part? This level of segmentation used to require expensive manual research or consulting projects. Now it can be automated at scale for pennies per classification.
Step 6: Set up personalized outreach sequences
The final step in the automated qualification workflow is connecting enriched, scored, and segmented data to the outreach process.
Using the segments and qualification scores from the system, dynamic outreach sequences can automatically adjust based on:
- Industry segment and sub-segment
- Company size and maturity
- Specific pain points indicated by their technology stack
- Recent trigger events (funding, growth, leadership changes)
- Engagement history with content
Each sequence should have unique messaging that speaks directly to the prospect's specific situation. For example, a sequence for enterprise healthcare providers might focus on compliance and integration with existing systems, while a sequence for tech startups might emphasize quick implementation and scalability.
Beyond just customizing message content, enriched data should inform:
- Channel selection (email, LinkedIn, phone, etc.)
- Outreach timing and frequency
- Content and resources shared
- Call-to-action type (demo, consultation, content)
- Sales rep assignment based on expertise
This level of personalization at scale is only possible with robust data enrichment and automated qualification. It transforms generic spray-and-pray outreach into precisely targeted communications that feel personally crafted for each recipient.
Using AI for advanced qualification and segmentation
AI is revolutionizing how enriched data is processed and acted upon for lead qualification. Here's how AI can be leveraged within platforms like Databar.ai to take qualification to the next level.
Custom industry classification with AI
As mentioned earlier, standard industry classifications often don't align with how markets are segmented. AI can analyze company descriptions, website content, and social media to place prospects into custom-defined segments.
Within Databar.ai, an AI-powered workflow can:
- Pull company information from multiple sources
- Analyze the text to understand what the company does
- Classify the company into predefined industry segments
- Further categorize them into sub-segments
- Assign specific messaging templates based on their classification
For example, if "Marketing Technology" is defined as a target segment with sub-segments like "Analytics," "Content Management," and "Automation," the AI can analyze a company's website and determine which category best fits them.
This goes far beyond keyword matching. The AI understands context and can distinguish between a company that uses marketing automation (a potential customer) and one that develops marketing automation software (a competitor).
Intent signal analysis with AI
Raw intent data can be overwhelming – thousands of data points showing website visits, content consumption, and research activities. AI can process these signals to identify meaningful patterns and buying intent.
For example, an AI model might determine that companies who visit a pricing page three times in a week and also download a comparison guide are 4x more likely to purchase than those who only read blog content.
Databar.ai can ingest intent data from multiple providers, apply machine learning to identify the most predictive patterns.
Predictive lead scoring with machine learning
Traditional lead scoring assigns fixed point values to different attributes. Predictive scoring uses machine learning to dynamically adjust these values based on actual conversion data.
As the sales process unfolds, the system learns which factors truly predict successful deals. Maybe company size matters less than thought, while specific technology usage is more predictive than expected. The model adjusts automatically, improving qualification accuracy over time.
To implement predictive scoring in Databar.ai:
- Start with an initial scoring model based on assumptions
- Connect closed-won and closed-lost data from the CRM
- Enable the machine learning module to analyze conversion patterns
- Review and apply the updated scoring weights
- Set up regular retraining to continuously improve the model
This approach eliminates the guesswork from lead scoring, replacing assumptions with data-driven insights about what really drives conversions in the business.
Measuring the impact of automated qualification
Implementing automated qualification only matters if it improves business results. Here's how to measure the impact and continuously refine the approach.
Key metrics to track
Qualification accuracy – What percentage of leads marked as qualified by the system actually engage with sales or convert? This measures how well the criteria predict actual buying behavior.
Time to qualification – How quickly can the system accurately qualify incoming leads? Faster qualification means quicker follow-up and higher conversion rates.
Coverage rate – What percentage of leads can be fully enriched and scored? Low coverage might indicate gaps in data sources.
Conversion rate by score band – How do conversion rates differ between leads scored 80-100 versus 60-80 versus 40-60? This helps validate scoring thresholds.
Cost per qualified lead – How has automated qualification affected the overall cost to generate a qualified lead? This measures ROI on data enrichment investment.
Continuous improvement process
Automated qualification isn't a set-it-and-forget-it solution. Implementing a regular review cycle to analyze results and refine the approach makes sense:
- Monthly data audit – Check for gaps or inaccuracies in enriched data.
- Quarterly criteria review – Analyze which qualification criteria best predicted actual sales.
- Segment performance analysis – Determine which custom segments convert best and why.
- A/B testing of thresholds – Experiment with different qualification thresholds to optimize resource allocation.
- Feedback loop with sales – Gather input from sales on the quality of qualified leads and missing data points.
Using these insights to continuously refine qualification criteria, data sources, and scoring models is valuable. The beauty of an automated system is that improvements can be implemented at scale, immediately benefiting all future qualification decisions.
Common pitfalls in automated qualification (and how to avoid them)
Even with the right tools, companies can stumble when implementing automated qualification. Here are the most common mistakes and how to prevent them:
Data tunnel vision – Relying too heavily on a single type of data (usually firmographics) while ignoring other signals. Avoid this by creating a balanced qualification model that incorporates multiple data types – firmographic, technographic, intent, and engagement data.
Overcomplicating scoring models – Creating excessively complex qualification criteria that are difficult to maintain and explain. Start with a simpler model focusing on the 5-7 most predictive factors, then expand gradually as results are validated.
Ignoring data decay – Failing to refresh enriched data regularly, leading to decisions based on outdated information. Implement automated data refreshes through Databar.ai based on the typical change frequency for each data type.
Neglecting sales feedback – Building qualification models without input from sales on what truly indicates a qualified lead. Create a structured feedback loop where sales can easily flag qualification issues and suggest improvements.
Binary thinking – Treating qualification as a yes/no decision rather than recognizing different degrees of readiness. Implement a tiered qualification system with different follow-up approaches for each level, from immediate sales contact to longer-term nurturing.
Set-and-forget mentality – Implementing qualification automation and never revisiting or refining the criteria. Schedule regular reviews to analyze qualification accuracy and update models based on actual results.
By avoiding these pitfalls, a qualification system can be built that continuously improves, delivering ever-more-accurate results as it ingests more data and learns from outcomes.
Conclusion
Automating lead qualification and segmentation with enriched data is essential for sales success in today’s crowded marketplace. By combining firmographic, technographic, intent, engagement, demographic, and behavioral data, companies can build a detailed, dynamic view of each prospect. This enables precise lead scoring, custom industry segmentation, and personalized outreach that truly resonates. Leveraging platforms like Databar.ai and AI-driven workflows makes this process scalable, accurate, and adaptable. The result: higher conversion rates, shorter sales cycles, and more efficient use of sales resources. Avoid common pitfalls by balancing automation with human insight and continuously refining your models based on real-world feedback. With the right approach, automated qualification transforms generic outreach into targeted conversations that turn leads into loyal customers faster and more consistently.
Related articles
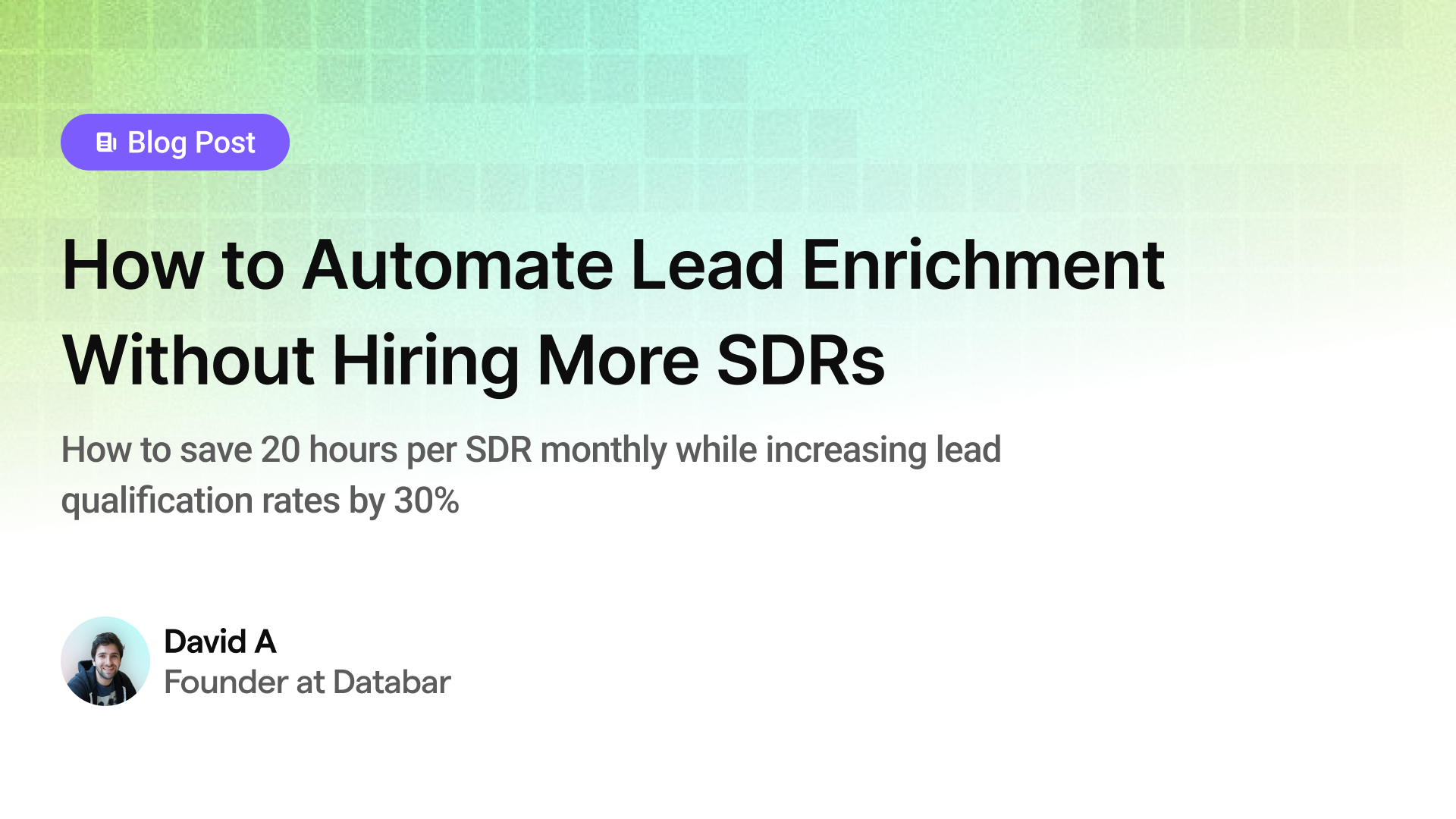
How to Automate Lead Enrichment Without Hiring More SDRs
How to save 20 hours per SDR monthly while increasing lead qualification rates by 30%
by Jan, April 19, 2025
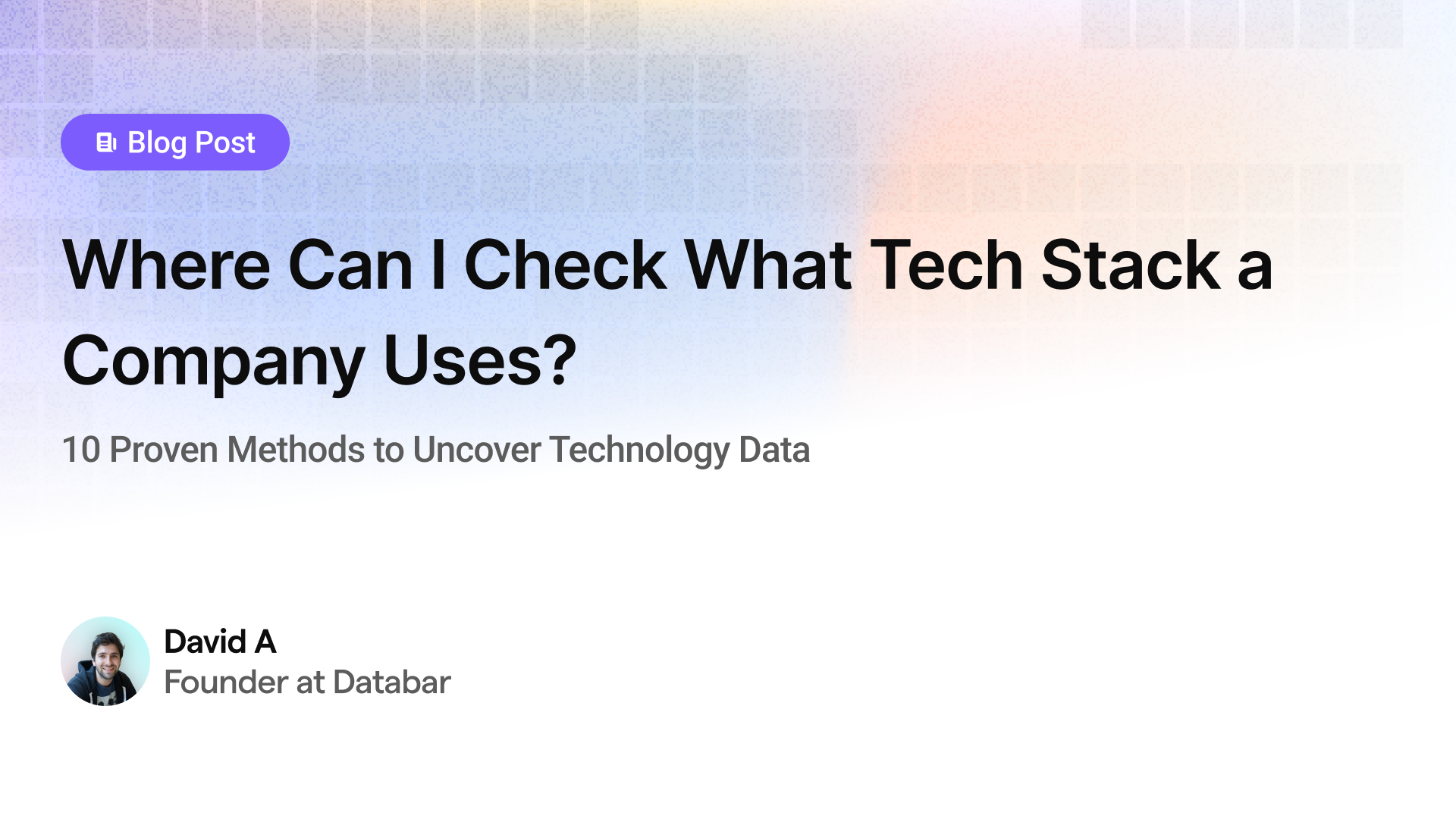
Where Can I Check What Tech Stack a Company Uses?
10 Proven Methods to Uncover Technology Data
by Jan, April 18, 2025
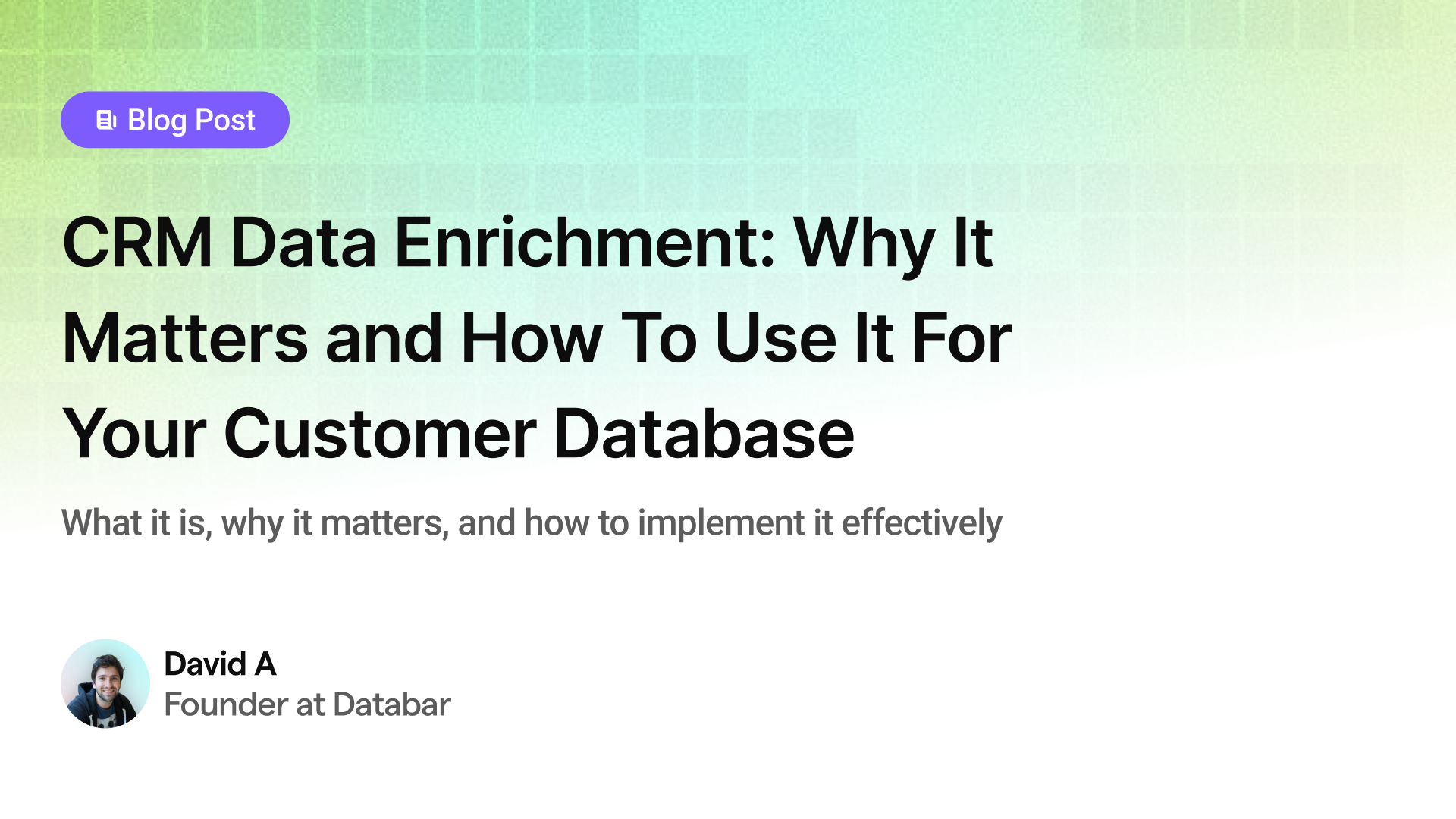
CRM Data Enrichment: Why It Matters and How To Use It For Your Customer Database
What it is, why it matters, and how to implement it effectively
by Jan, April 18, 2025
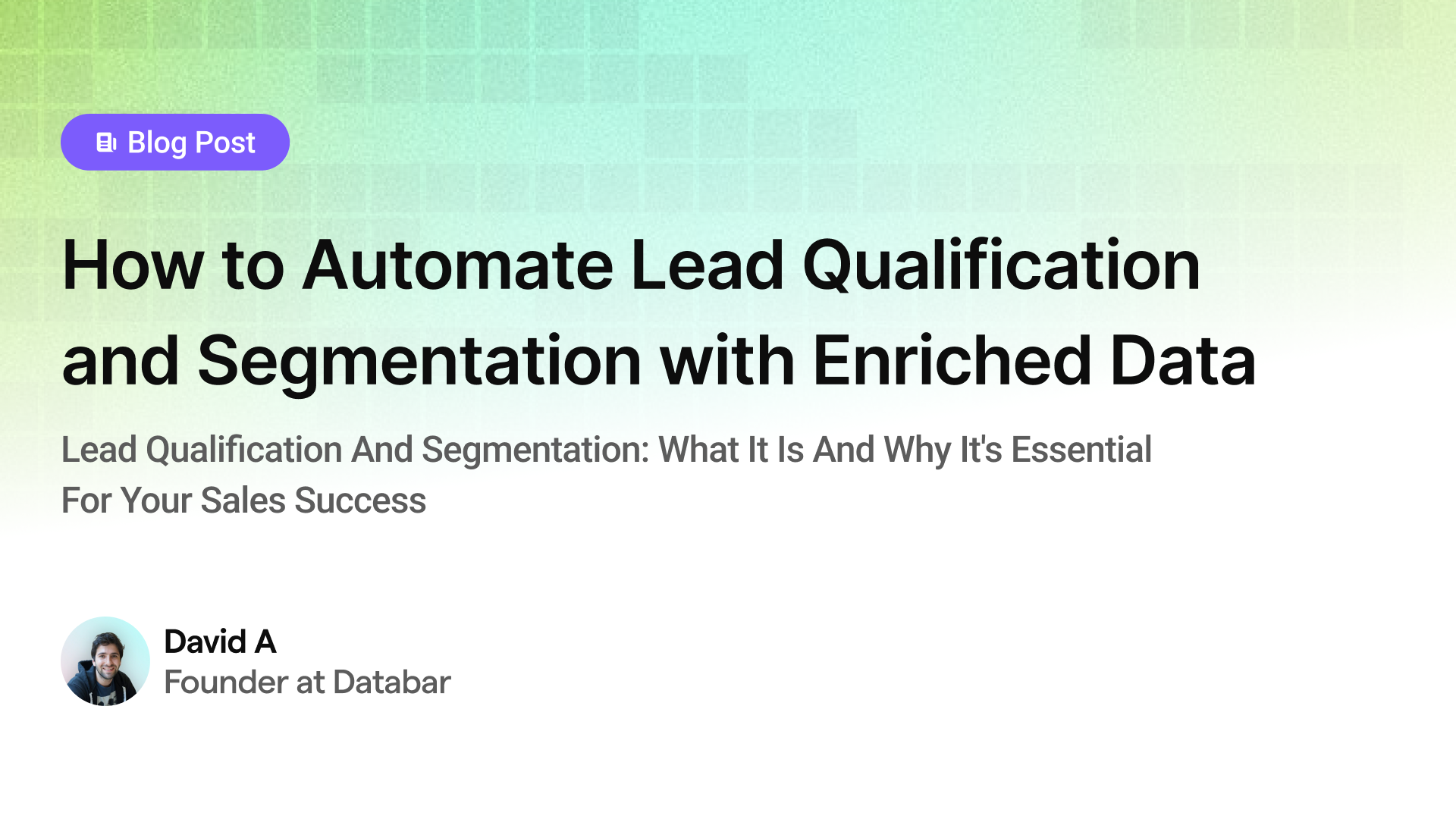
Waterfall Enrichment: How to Boost Your CRM Data Quality and Coverage by 3x
Eliminate data gaps and boost sales performance with multi-source enrichment strategies
by Jan, April 16, 2025
Your guide to APIs without code
Subscribe to our newsletter to receive updates and exclusive information on trends and updates in APIs.